🚀 Exciting News in Healthcare Innovation! 🚀
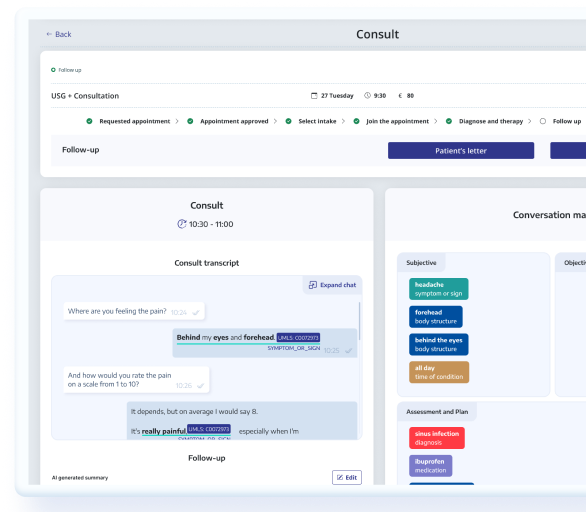
As healthcare professionals, we understand the challenges of navigating through vast amounts of textual data in electronic health records (EHR). It's time-consuming and often takes away from the personalized patient care we strive to deliver. Nonetheless, at HealthTalk, we're actively developing a solution to address this challenge!
A groundbreaking study conducted by researchers at Stanford confirms; the potential of large language models (LLMs) in revolutionizing clinical text summarization was explored. By applying innovative domain adaptation methods to eight LLMs across six datasets and four distinct clinical summarization tasks, including radiology reports and progress notes, they aimed to alleviate the documentation burden on clinicians.
Their findings? The same as ours...
Not only do LLMs provide summaries that surpassed human-generated ones in terms of completeness and correctness, but they also demonstrated a level of conciseness that can significantly enhance clinical workflows. This breakthrough shows that integrating LLMs into our processes could lead to more efficient allocation of our time, empowering us to focus on what truly matters: personalized patient care and the human aspect of medicine.
Moreover, their study delved into the nuances of LLM adaptation methods and their impact on performance, shedding light on the most effective strategies for leveraging these powerful tools in healthcare settings.
With the potential to reduce documentation load and improve patient outcomes, LLMs represent a promising avenue for innovation in healthcare. By embracing these advancements at HealthTalk, you as healthcare provider can streamline tedious tasks and dedicate more time to building meaningful patient relationships and delivering tailored care.
Join us in embracing the future of healthcare innovation! Let's work together to leverage technology in enhancing the way we deliver care. Read the full research paper here.